MNIST 데이터는 0 ~ 9 까지 손글씨로 이루어진 이미지 데이터 셋이다.
MNIST Yann LeCun 웹사이트에서 제공해주고 설치가 가능하다.
1. MNIST Dataset 설치
MNIST in CSV
MNIST in CSV Here's the train set and test set. The format is: label, pix-11, pix-12, pix-13, ... where pix-ij is the pixel in the ith row and jth column. For the curious, this is the script to generate the csv files from the original data. def convert(img
pjreddie.com
해당 홈페이지에서 train set과 test set을 미리 설치해 둔다.
2. Jupyter Notebook 실행
Anaconda에서 Jupyter Notebook을 설치하고 실행하면 아래와 같이 파일들이 나와있을 것이다.
해당 파일들의 경로는 PC에서 C:\{사용자이름}\user\
에 존재한다.
1번에서 다운받은 데이터셋을 해당폴더에 옮겨준다.
글쓴이는 source에서 작업을 해서 source 폴더로 옮겨줬다.
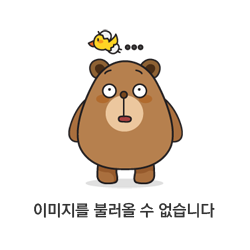
화면 오른쪽에 New를 클릭하고 Python 3을 클릭하면 프로젝트가 생성된다.
프로젝트가 생성되었으면 준비 끝!
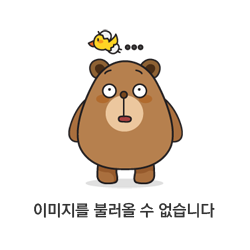
3. MNIST 실습
# 3개층의 신경망으로 MNIST 데이터를 학습
import numpy
import scipy.special
import matplotlib
%matplotlib inline
#신경망 클래스 정의
class neuralNetwork:
def __init__(self, input_nodes, hidden_nodes, output_nodes, learning_rate):
# 입력, 은닉, 출력 계층의 Node 수 설정
self.inodes = input_nodes
self.hnodes = hidden_nodes
self.onodes = output_nodes
# 가중치 행렬 설정
self.wih = numpy.random.normal(0.0, pow(self.inodes, -0.5), (self.hnodes, self.inodes))
self.who = numpy.random.normal(0.0, pow(self.hnodes, -0.5), (self.onodes, self.hnodes))
# 학습률
self.lr = learning_rate
# 시그모이드 활성화 함수
self.activation_function = lambda x: scipy.special.expit(x)
# 신경망 학습
def train(self, inputs_list, targets_list):
# 입력과 타겟 리스트를 2차원 행렬로 변환
inputs = numpy.array(inputs_list, ndmin=2).T
targets = numpy.array(targets_list, ndmin=2).T
## 순전파
# 입력 계층 - 은닉 계층
hidden_inputs = numpy.dot(self.wih, inputs)
hidden_outputs = self.activation_function(hidden_inputs)
# 은닉 게층 - 출력 계층
final_inputs = numpy.dot(self.who, hidden_outputs)
final_outputs = self.activation_function(final_inputs)
## 역전파
# 출력 계층 오차 = 실제값(정답) - 계산 값
output_errors = targets - final_outputs
# 은닉 계층 오차 = 출려 계층 오차를 역전파
hidden_errors = numpy.dot(self.who.T, output_errors)
# 가중치 update
self.who += self.lr * numpy.dot((output_errors * final_outputs * (1.0 - final_outputs)), numpy.transpose(hidden_outputs))
self.wih += self.lr * numpy.dot((hidden_errors * hidden_outputs * (1.0 - hidden_outputs)), numpy.transpose(inputs))
# 신경망에 질의
def query(self, inputs_list):
inputs = numpy.array(inputs_list, ndmin=2).T
hidden_inputs = numpy.dot(self.wih, inputs)
hidden_outputs = self.activation_function(hidden_inputs)
final_inputs = numpy.dot(self.who, hidden_outputs)
final_outputs = self.activation_function(final_inputs)
return final_outputs
input_nodes = 784
hidden_nodes = 100
output_nodes = 10
learning_rate = 0.1
n = neuralNetwork(input_nodes, hidden_nodes, output_nodes, learning_rate)
# MNIST 학습 데이터 불러오기
training_data_file = open("mnist_dataset/mnist_train_100.csv", 'r')
training_data_list = training_data_file.readlines()
training_data_file.close()
epochs = 1
for e in range(epochs):
for record in training_data_list:
all_values = record.split(',')
inputs = (numpy.asfarray(all_values[1:]) / 255.0 * 0.99) + 0.01
targets = numpy.zeros(output_nodes) + 0.01
targets[int(all_values[0])] = 0.99
n.train(inputs, targets)
#MNIST 테스트 데이터 불러오기
test_data_file = open("mnist_dataset/mnist_test_10.csv", 'r')
test_data_list = test_data_file.readlines()
test_data_file.close()
all_values = test_data_list[0].split(',')
print(all_values[0])
image_array = numpy.asfarray(all_values[1:]).reshape(28,28)
matplotlib.pyplot.imshow(image_array, cmap="Greys", interpolation='None')
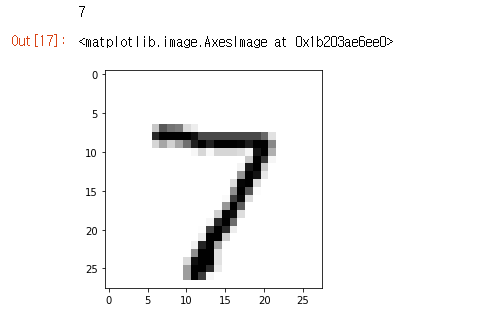
이러한 결과가 나오게 된다.
홍콩 과기대 김성훈 교수님의 모두를 위한 Deep Learning 강좌를 보고 공부했으며 아래 사이트에서 강의 자료 및 강의 영상을 확인 할 수 있다.
[참고 자료] http://hunkim.github.io/ml/
[참고 자료] https://github.com/hunkim/DeepLearningZeroToAll
'Programming > Python' 카테고리의 다른 글
[Python] Lambda(람다) 사용법 (0) | 2021.12.24 |
---|